Sample size matters: do people pay attention to how big a study is?
Journalists and business leaders need to be careful about misleading others when it comes to broadcasting research findings based on small studies
Early in the COVID-19 pandemic, pharmaceutical and biotechnology company Moderna (MRNA) reported its experimental vaccine was successful in eight volunteers. While only a small group of healthy volunteers were tested, journalists quickly spread the news, and Moderna's share price went up by 20 per cent. Just hours after announcing the trial's success, Moderna sold 17.6 million shares to the public, raising US$1.3 billion. While Moderna, and several of its top executives, profited off the back of the boom, some critics say it overstated the significance of the vaccine trial and manipulated the market. Examples like these demonstrate that most people don't overthink the significance of a study's size when making assumptions from what they read online.
When presented with qualitative research findings in the media, evidence shows people are quick to jump to conclusions, even when the study’s sample size is very small. And for anyone who has lived through the COVID-19 pandemic, this probably comes as no surprise.
A research finding is only good if it’s statistically reliable. So while the study doesn’t have to test the whole population to come up with reliable findings, the sample size (e.g., number of participants or observations) should still be significant enough to represent the target population and have statistical power. Otherwise, it can lead to the spread of misinformation.
In a recent study of almost 4000 participants, Dr Siran Zhan, Senior Lecturer in the School of Management and Governance at UNSW Business School, finds people might not have the correct intuition as to what counts as evidence, making it difficult to correctly use statistics and research evidence to guide their inferences and decisions. The paper, Relative Insensitivity to Sample Sizes in Judgments of Frequency Distributions, written by Dr Zhan and her co-author, Dr Krishna Savani, Professor of Management at the Department of Management and Marketing at The Hong Kong Polytechnic University, shows people ignore the sample size in their judgments and decisions and tend to be unduly confident in conclusions drawn from studies with as few as three participants.
“What surprised us was that when we examined samples of university-level statistics students and seasoned senior executives who are supposedly trained in their education or professional work to make judgments and decisions according to sound statistical principles, they ignored the sample size just as much as the public. It is especially appalling to think many important businesses and public policy decisions might have been made based on unreliable results from small samples,” she says.
The good news? The researchers also tested a way to prevent the spread of misinformation.
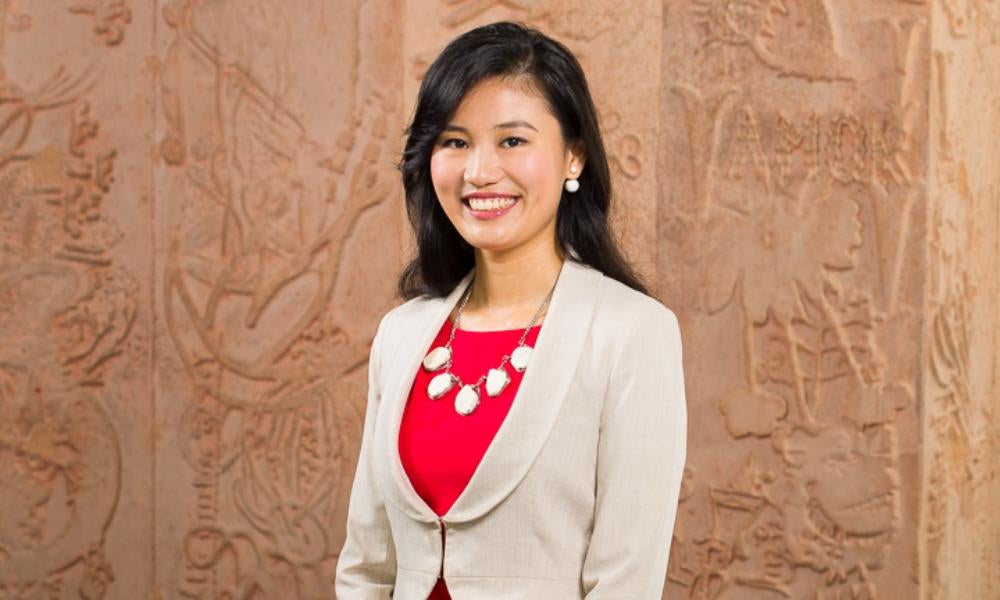
What is a sample size, and why is it important?
In the study, six experiments involving a total sample of 3914 respondents test whether people pay attention to variations in the sample sizes, which vary by one or two orders of magnitude. The findings reveal people pay minimal attention to variations in the sample size by a factor of 50, 100, and 400 when making judgments and decisions based on a single sample. “In other words, people’s general tendency to be unduly confident in conclusions drawn from tiny samples is incommensurate with statistical principles and can lead to poor judgment and decisions,” explains Dr Zhan.
“Even with a sample size of three, participants’ mean confidence level was 6.6 out of 10, indicating that people have pretty high confidence in data from incredibly small samples, consistent with prior research,” explains Dr Zhan. “As researchers, we realise that the same finding is much more believable from a sample of 3000 than from a sample of 30. However, shockingly, the general population does not appear to share this intuition.”
With the increasing spread of online disinformation and misinformation, making judgements about what we’re presented with in the media is becoming increasingly important. “With the proliferation of statistics in the news media and in organisations that call for evidence-based decision-making, the current findings indicate that people might not have the correct intuition as to what counts as evidence, making it difficult for them to correctly use statistics and research evidence to guide their inferences and decisions,” explains Dr Zhan.
Read more: Google shouldn't subsidise journalism, but the government could
What’s an appropriate sample size?
Is there such a thing as the right sample size? “Bigger is generally better, statistically. This is because the mean result from any sample is pulled or biased by outliers. The only issue is the cost of time and money to collect data from a very big sample. Therefore, a trade-off must be made based on sound statistically ground so that we work with a statistically reliable yet realistically feasible sample size.” explains Dr Zhan.
There is not a one-size-fits-all magical number here. Instead, it depends on how large the real effect is (e.g., whether a new design improves user experience by 10 per cent or 50 per cent) and how confident you want to be of your conclusion (e.g., generally, the more consequential a misestimate, the more confident you want to be). In other words, the margin of error should be fairly low.
“Generally, the larger the sample size, the more reliable the results will be. But of course, larger sample sizes come at the cost of time and money; thus, no one can or should aim for indefinitely large sample sizes. It might not be so straightforward for non-statistically trained individuals; we recommend providing more statistical advice in a layperson language, a practice currently lacking.”
When the sample size is small (e.g., 30), any outlier has a much stronger effect on the mean, making your mean less reliable than when the sample size is large (e.g., 3000). Put another way, when you estimate an effect from a sample (e.g., 500 customers), you are always trying to generalize your result to a population (e.g., your 13,974 existing customers), which in reality, is too large for you to thoroughly study. When your sample size increases, your sample gets closer to the population, which means fewer estimation errors,” she says.
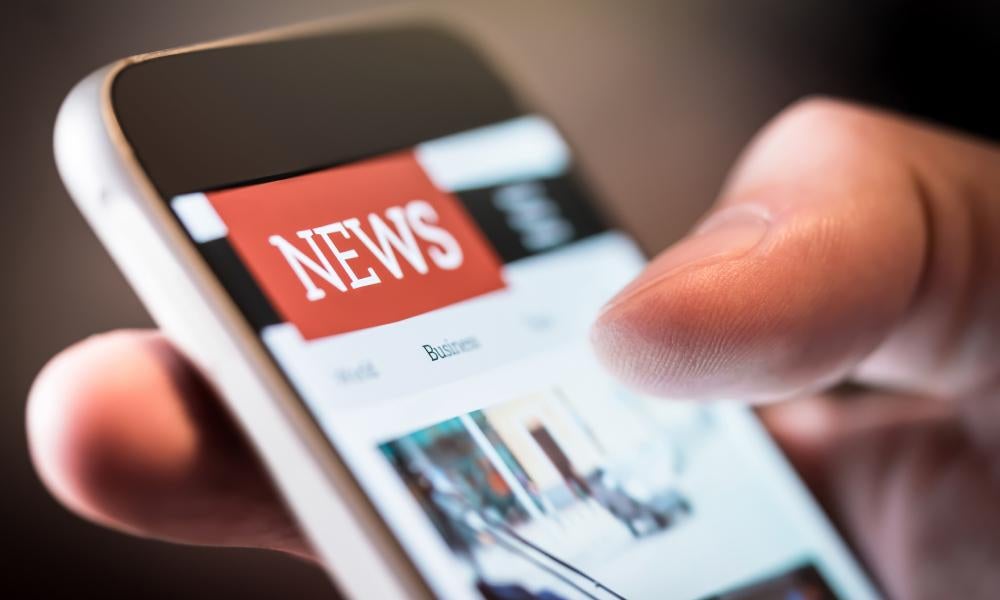
Study design to help prevent the spread of misinformation
Judgements and biases regarding research design and methodology don’t just affect what we read in the media; these judgements permeate almost every aspect of our lives, from public policies to workplaces. “Organisations evaluate employee performance based on a limited time window or a small number of projects (e.g., monthly sales record or past three projects). In these cases, entrepreneurs and managers need to understand that their findings, however substantive, may not be reliable if they were drawn from small samples,” explains Dr Zhan.
Therefore, Dr Zhan’s research holds important implications for managers, entrepreneurs, and policymakers who often use results from samples (sometimes tiny samples) to inform critical decisions. To improve decision quality, Dr Zhan suggests that all statistics must be accompanied by statistical inferences (whether in the null hypothesis significance testing or the Bayesian frameworks), along with ‘layperson interpretations’ of the statistical inferences.
Indeed, Dr Zhan’s research finds that reducing people’s insensitivity to sample sizes is possible by providing people with a layperson interpretation of the strength of evidence statistics. In other words, a simple intervention can help reduce people’s insensitivity to sample sizes – by providing people with a layperson’s interpretation of the strength of evidence statistics. She also recommends more statistical advice be provided to aid their interpretation of findings and decision-making.
“For example, the Environmental Working Group provides a searchable online database with information on skincare product safety (example here) on two primary scores: The strength of an effect (i.e., the hazard score) and the strength of evidence (i.e., data availability). The data availability information is equivalent to the strength of evidence information that we are advocating here,” explains Dr Zhan.
Subscribe to BusinessThink for the latest research, analysis and insights from UNSW Business School
Entrepreneurs and managers must understand that their data collection methods and research findings (for example, in market research), however substantive, may not be reliable if they were drawn from small samples. “We recommend more statistical advice (i.e., a layperson interpretation of the strength of evidence statistics) to be provided to aid their interpretation of findings from samples and, ultimately, decision-making,” she says.
But what about consumers? “Consumers generally do not read research articles directly. Research reports generally reach consumers through product information, news, and popular books. Therefore, we recommend that the strength of evidence statistics be presented alongside data availability information.
“Consumers should be educated to question any claims unless there is strong evidence (i.e., a large amount of independent research involving large sample sizes). But educating consumers is difficult; more importantly, we think the burden must be placed on businesses and content publishers,” she says.
Dr Siran Zhan is an Assistant Professor in the School of Management at UNSW Business School. In her research, Dr Zhan investigates the individual (e.g., identity and cognitive biases) and social (e.g., culture and diversity) factors important to creative and entrepreneurial processes. For more information, please contact Dr Zhan directly.