Striving for safe AI in a fast-evolving business landscape
How should boards and executives prioritise AI risk literacy, compliance frameworks and governance standards to navigate evolving regulatory demands?
The risks associated with artificial intelligence (AI) and other technology can be esoteric and overly complicated, but it remains critical for organisations to stay well-versed in them as AI becomes increasingly embedded in business.
As Carl Fernando, Managing Director of Canda Consulting, emphasised during a recent conversation on cyber and climate risk hosted by AGSM @ UNSW Business School, it’s impossible to manage risk without understanding it. As a result, boards and executives should prioritise improving foundational literacy, awareness and training around AI and its associated risks, he said, noting that he has seen these lacking in many organisations.
As part of the Let’s Talk About Risk online symposium, Paul Vorbach, Founding Managing Director of AGSM partner AcademyGlobal, interviewed Mr Fernando about governance and ethics in AI, exploring pressing challenges and emerging trends in risk management before an audience of senior executives and risk professionals. They noted that there are critical and rapidly evolving challenges in the realm of cybersecurity and technology, making it essential that professionals and leaders know how to navigate the complex landscape.
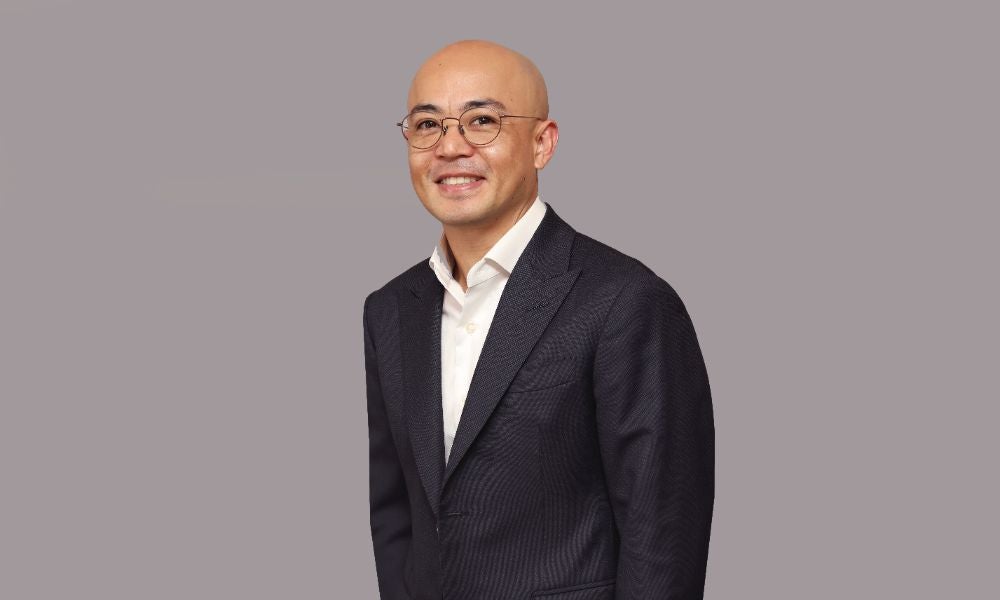
Understanding AI
Mr Fernando first recommended thinking about risk through “three lenses or concepts of AI”, beginning with a taxonomical question: what are the different types of AI that exist?
“If you think about the word ‘transport’, which is really broad – there are cars, buses, trains, boats, et cetera – that’s similar to AI, and there are very different types,” he said. “There are different pros and cons with AI capabilities and, indeed, different risks and opportunities. You want to understand what type of AI your organisation will want to work with.”
The second lens to apply is the concept of the components of the AI itself. “If you break down each of the different types – such as machine learning, natural language processing, large language models like ChatGPT – all of them have common elements,” he said.
Read more: How AI is changing work and boosting economic productivity
“You can think about the three main components of AI as a process, beginning with the input – what is it being trained on? Right at the centre is the model itself. And then, after you start using it in production, you’ve got outputs. If you think about those three components, that’s where you’ll start to see the sources of risk.”
Finally, he said, it’s essential to “understand AI in the form of how it can arrive to you in software” – for instance, a standalone AI (such as GPT) to be engaged with on its own or an embedded AI (such as Copilot in the Microsoft Suite). “How it arrives for your staff and how you use it will be very different in terms of the risk profile, as well.”
Unique risk profile
Some of the main risks unique to AI involve the three central components of input, algorithm/model and output, Mr Fernando said.
Input risks include the provenance of the data the model is being trained on – that is, the source and recency of the data, and whether it’s from the World Wide Web or from internal data – and the risk of data bias. “How has the model been trained; how biased is the data itself?” And there are different types of bias, each presenting further risks. The magnitude of bias is another challenge.
As for the AI model itself, there are unique risks around validation and explainability, according to Mr Fernando. “Validation in the traditional sense is whether the model is performing as intended,” he said. Because of AI’s continuously learning nature, this process must be dynamic, with validation carried out periodically and consistently.
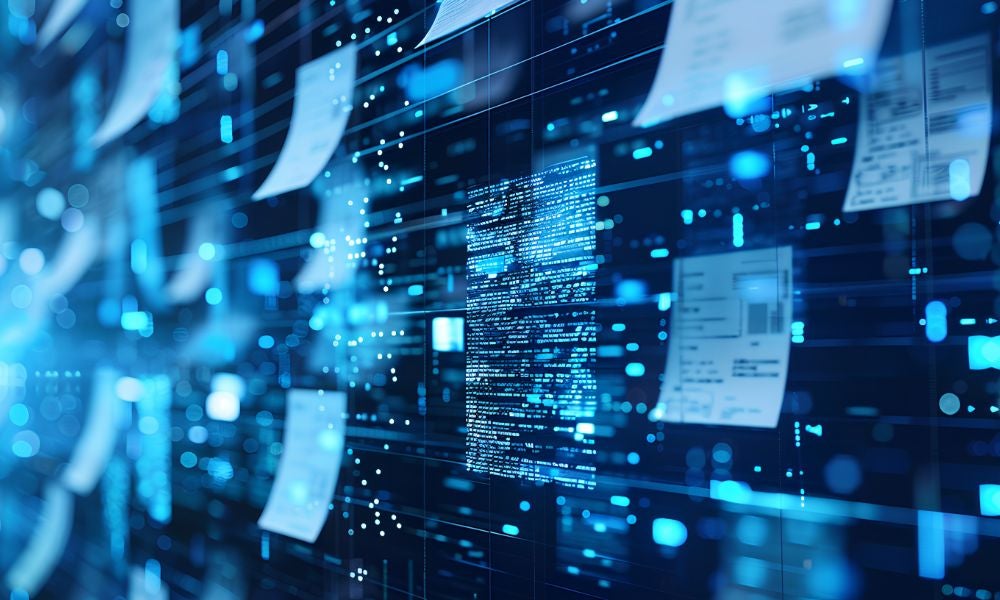
Explainability is increasingly becoming the subject of attention, with regulations and audit requirements mandating organisations using AI be able to explain how the AI arrives at its decisions and recommendations. “Finally, there are traditional risks such as privacy and security that are being augmented or even exacerbated by AI,” Mr Fernando said. “This is all really critical for organisations to understand.”
Compliance and regulation
Generally, the compliance and regulatory landscape has become dynamic and fast-moving and should be a focus for organisations as they develop their risk frameworks, according to Mr Fernando. He noted that the EU AI Act, enacted this year, was the “first mover from a comprehensive AI regulation perspective,” adding that the law is “quite general in terms of how it’s going to govern AI use”.
There are also more specific laws; for instance, in the US, the state of New York has adopted requirements governing the use of AI in employment recruitment. “I think we’re going to see a combination of this specific, targeted application of laws with the more general and comprehensive approach.”
Read more: Resilience in AI leadership: In conversation with Stela Solar
Moreover, compliance is not just a concern for larger organisations that are more likely to be bound by these laws. “There are ethical AI principles that are now being espoused by governments and NGOs [non-governmental organisations] across the globe,” Mr Fernando said, pointing to principles and frameworks published by the OECD and by the World Economic Forum, as well as local government frameworks.
“I think you’ll start to see better practice being espoused for responsible and ethical adoption of AI in addition to the laws and regulations,” he said. “The good thing is that we’re starting to see some common threads through these. Things like the laws and better-practice principles both requiring human oversight – being really clear that you can’t just have an autonomous AI making decisions – and that you then want to start thinking about the wider group of stakeholders when you’re doing risk and impact assessment. The laws and these better-practice principles are saying the same thing.
“There are going to be wider impacts from AI than just to the organisation itself,” Mr Fernando said. “We’re talking about individuals and groups of individuals in society who could be impacted, so the assessment of risk and impact is something that’s going to be clearly looked at.”
Leaning on standards
Finally, organisations must have an acceptable use policy, including training and upskilling staff and ensuring the appropriate policies are in place. “You can’t just ignore your accountabilities as an organisation and have a blanket ‘you can’t use AI’ type of approach; you’ve got to start to think about how to govern this in a more nuanced and appropriate way,” Mr Fernando said.
Subscribe to BusinessThink for the latest research, analysis and insights from UNSW Business School
He advised business leaders to start thinking within the context of existing standards. “I’ll admit that these aren’t going to be a panacea; there’s no such thing as a silver bullet,” he said. “But they will at least start to guide us on what we need to do to augment our existing risk management practices and how we need to think about governance practices.”
These include standards from the International Organization for Standardization (ISO), such as ISO 42001, the new AI management system standard, and ISO 23894, which will augment ISO 31000 with AI-specific considerations. In addition, the US National Institute of Standards and Technology now has an AI Risk Management Framework to support other NIST standards for the information technology field.
“These standards will really start to give us a little bit of a feel for what’s appropriate” in ethical AI and compliance considerations, Mr Fernando said. “I think more and more professionals are now coming online to support organisations with responsible and ethical AI adoption, which is really pleasing,” he concluded.