How to innovate responsibly in an era of AI democratisation
Navigate the complexities of AI implementation while mitigating associated risks and building a robust foundation for responsible AI practices within your organisation
As AI moves from proofs of concept to enterprise solutions and from facilitating work to automating it, risks like over-reliance and failure to embed mean forward-thinking businesses should have a clear plan for implementing AI.
But this moment also translates to an opportunity for forward-thinking businesses that properly integrate responsible AI, according to PwC Australia's Director of Responsible AI, Charles Lee, and Director of AI Assurance, Rob Kopel. In a recent interview with Dr Lamont Tang, Director of Industry Projects at AGSM @ UNSW Business School, Mr Lee and Mr Kopel urged businesses and leaders to increase their “AI fluency”, upskilling and enabling their people for augmented output in an AI-driven enterprise.
Responsible AI began as a framework for building guardrails around bespoke AI models and defining risk in an AI context. In recent years, responsible AI has moved from being niche to “no longer just a thing data scientists do, but something everyone needs to be cognisant of,” Mr Lee explained. The shift results from the advent of generative AI and large language models (LLMs) like those behind ChatGPT, which have democratised AI and “risen all tides for responsible AI – it’s becoming a lot more mainstream”, he said.
But AI’s enhanced impact makes it critical that we act responsibly with it. Moreover, there are growing indications that regulators are taking a greater interest in AI and the responsibilities it creates for businesses. “I think it’s interesting to see even regulators and legislative bodies taking a look backwards now and asking, 'How have you been managing the risks of your existing AI?'" Mr Kopel observed.
As a result, evolving standards – notably the US National Institute of Standards and Technology (NIST) ’s January 2023 release of an AI Risk Management Framework – provide companies with fundamental principles to follow as they work out how to implement AI responsibly. “At that step-down, there’s also been a lot of growth, both in terms of government legislative-led frameworks and in terms of open-source and commercially led frameworks,” Mr Kopel said.
Mr Lee added that before the NIST framework, responsible AI had primarily aimed to define the risks in AI, such as bias, interpretability and robustness, and apply guardrails and controls to bespoke models. “With the advent of regulation being passed and that trickling down, I think we’re in a really interesting time in Australia,” he said. “And now, corporations are expected to lead on it.”
Over-reliance and other risks
Use cases play a significant role in companies’ efforts to define AI policies in this landscape, as does making appropriate organisational preparations. “From a component point of view, I think every organisation today should have an AI policy, a risk assessment framework and some control standards among the end-users and the data science teams who consume and develop AI systems,” Mr Lee said.
A big challenge in use cases is the risk of the “humans in the loop” becoming negligent due to AI augmentation. “We’ve seen quite a few times organisations taking an existing process, adding AI to accelerate a piece of that process and not closely monitoring it,” he said.
Read more: Resilience in AI leadership: In conversation with Stela Solar
According to Mr Lee, these challenges are part of the iterative nature of technological advancements in AI. “They’re taking the learnings from those use cases and applying them forward and asking, ‘How are we going to facilitate more advanced and more complex use cases when we know that the silver bullet isn’t a human in the loop every time?’”
And while there is a “broad spectrum of mitigation efforts” for these challenges, sometimes a different approach is necessary in the highest-risk areas. For instance, in the example of medicine, where “we’re dealing with people’s lives, families – the stakes are high”, Mr Kopel said. He cited a consequent push for a model of AI in medicine as an “unbiased estimator” for comparing and analysing actual results.
“Rather than necessarily automating that work, we can use it as a secondary layer of quality assurance or verification, until we’re at that level where it’s achieving significantly greater precision than a human,” he said.
Building trust is key
Mr Kopel said there is a massive gap between companies’ recognition of AI’s potential impact and their actual implementation of AI. He cited a global PwC survey showing that approximately 70 per cent of CEOs expect generative AI to change their business significantly over the next three years, but only around 30 per cent have started to apply it.
There’s a “whole spectrum of reasons” for this gap around industrialisation and putting generative AI into practice, but Mr Kopel said trust is a recurring factor. He pointed to cases in the media where organisations have implemented AI ineffectively, leading to reputational damage or internal resistance.
Ultimately, he said the main risks involve implementing AI responsibly and safely and building the proper controls to gain the trust required to deploy it. Mr Lee added that deploying a massive change like generative AI requires an operating model that fits the change. “How do you be an innovator in this space when being a fast follower isn’t enough?” he said. “And how do you completely revamp your operating model?”
Discussing his team’s experience, Mr Kopel said PwC “treated itself as client zero” when the firm first began devising its AI policy. “What we realised was that, when we started drafting our policy of, ‘here’s what you can use AI for, here’s what you can’t use AI for, and these are the risks, controls and considerations,’ – when we started working through this, it was best treated as an evolution of the existing policy frameworks that the firm already had, rather than standalone ‘net new’ risk considerations,” he said.
Australia’s existing legislative and regulatory framework, including the Privacy Act of 1988 and the Anti-Discrimination Act, already guides companies on “how to do business in the correct manner”, Mr Kopel said.
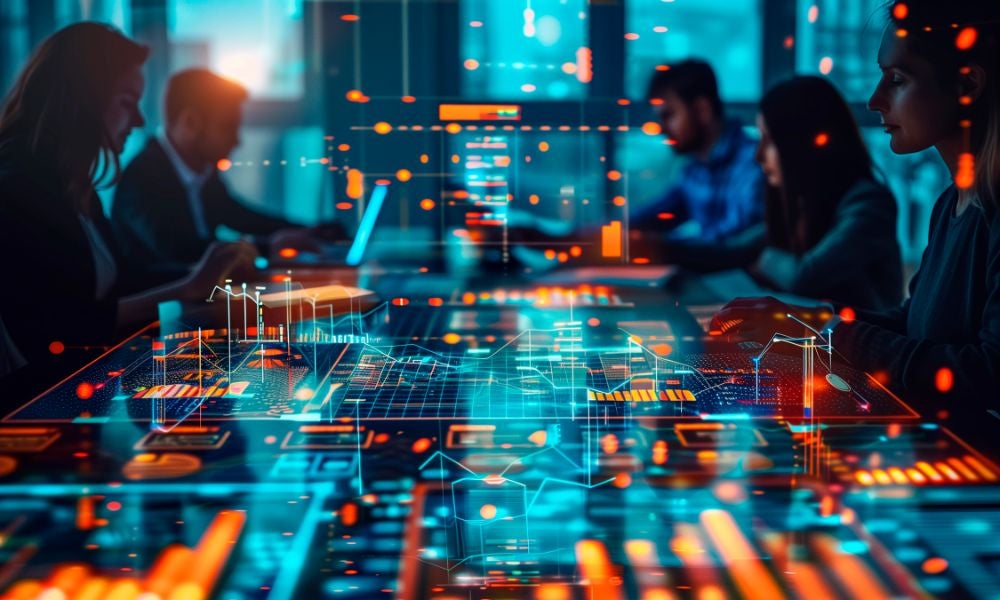
From policy to practice
Mr Kopel said the challenge most businesses face today is the transition from policy to practice. But this is also where he sees opportunity.
The vision in AI is a move from facilitation to automation, and many of the examples he sees of failure in the marketplace occur when companies “try to break this barrier”. Therefore, companies must understand the risk-benefit trade-off involved in deploying AI. “You’re going to have to have a very open discussion about that while you also take into account – and you really do have to make sure you take into account – the moral and ethical implications of whatever the use case is,” Mr Kopel said.
Mr Lee agreed that it’s a “big jump from AI facilitation to AI automation”, adding that the organisations that do well tend to “try a lot of back-office AI use cases so they get those learnings in terms of the gaps and misses and unknowns they didn’t think about when they developed those use cases in the first place.
“Instead of sitting in a room and thinking about the potential risks and what can go wrong, it’s sometimes more effective to try on yourselves; for example, in your back-office operations with safeguards in place, and taking those learnings and applying it to customer-facing areas,” Mr Lee added. “That’s probably the most efficient way to understand what the risks are for organisations, for their ecosystems and customers, and for society in general.”
Going enterprise
Mr Kopel proposed thinking of 2024 as a “year of industrialisation – going beyond proof of concepts and experimentation”, he said. ”Let’s actually build some things and drive real benefit out of them.”
He added that after a year of organisations exploring generative AI tools, “this year is where people have actually said, ‘We’re going to invest big, we’re going to adopt some of these platforms, try out all these different models, and start building teams who really understand these concepts. We’re going to start doing training and upskilling.’ I think that’s been the story for the year so far.”
As for the technology story, he noted that 2024 has seen an increase in consistency in LLMs’ ability to perform tasks. “That’s the real scale that a lot of people struggle with in organisations – first of all, how do you actually use a process when it fails one out of 20 times?” he asked.
“But as that starts improving, you can put more and more reliance on these systems, and you can actually use them in processes and not just where a human has to continually check every single output, where maybe that process doesn’t need to be 100 per cent accurate – it’s only an approximate process, so we can drive that benefit today.”
Mr Lee suggested that 2023 was the year of proofs of concept, a stage with “limited real risk” of harming an organisation’s ecosystem or customers. “As we move into making some of these generative AI solutions enterprise, I think a lot of organisations will have to revisit what their AI policy is and how they’re going to identify the controls for each use case, and how they’re going to roll that out from an enterprise point of view, how they’re going to test it, and how they’re going to put it into production and be customer-facing,” he said.
Subscribe to BusinessThink for the latest research, analysis and insights from UNSW Business School
Innovating responsibly
Achieving the balance of responsible innovation is “hard to do” and Mr Kopel said this is especially true in use cases where companies deploy generative AI across the entire business for everyone to apply to their workloads.
“There are so many unexpected consequences of that, and so it isn’t something where we build a policy once, set it and leave it set,” he said. “It’s something where we need continuous monitoring, continuous understanding and re-evaluation as you understand the different use cases and how your staff are applying it.”
Investing in safety and innovation allows an enterprise to “go faster, not slower”, Mr Lee noted. For one thing, employing the proper guardrails and feedback mechanisms enables companies to catch issues more quickly. “You can evolve your AI policy as you go along and make sure that it’s a lot more robust to handle the various things we didn’t foresee coming into this,” he said. “Prior to 2023, we didn’t think that AI was going to have such an accelerated impact on the way we think about business, the way we think about the use cases and the way we augment our processes.”
Ultimately, it’s essential that companies have both a technological and a business view of how they want to implement AI. “A forward-looking point of view is, how are you going to make your organisation more data-driven? And how are you going to put AI at the forefront of your agenda?” Mr Lee said. “[Those] that can get it across and embedded in their organisation are the ones that are going to have that comparative advantage and first-mover advantage in the space.”