How insurers can mitigate the discrimination risks posed by AI
Research reveals insurers can use fairness criteria alongside AI to minimise bias and discrimination risks
Artificial intelligence (AI) is changing the insurance industry. According to a global PwC survey, a quarter of insurance companies reported widespread adoption of AI in 2021, up from 18 per cent in 2020. Another 54 per cent said they were implementing AI and were looking to scale up. With AI set to become more deeply integrated within the industry, insurers must position themselves to respond to the changing business landscape. This means insurers must get smart about AI and its potential risks.
The use of AI can help insurers assess risk, detect fraud and reduce human error in the application process, but algorithms can also be biased. This means insurers' decisions can be based on misleading or biased data, which can lead to unfair discrimination against clients. While anti-discrimination laws exist, there are currently inadequate constraints on how insurers are allowed to use consumers’ data. On top of this, insurance pricing tools are complicated, and it can be difficult to work out where the biases occur. As insurance regulators around the world look to create new legislation to navigate this complex environment, insurers must find ways to ensure policy pricing is fair or face the repercussions of treating clients unfairly.
One way to ensure insurance policies are priced fairly is for insurers to adopt robust fairness criteria, says Dr Fei Huang, Senior Lecturer in the School of Risk and Actuarial Studies at UNSW Business School. In a recent study Anti-Discrimination Insurance Pricing: Regulations, Fairness Criteria, and Models, co-authored with a PhD student under her supervision Xi Xin, Dr Huang examines fairness criteria that are potentially applicable to insurance pricing and matches them with different levels of potential and existing anti-discrimination regulations and then implements them into a series of existing and newly proposed anti-discrimination insurance pricing models. The authors compare the outcome of different models, contributing to a deeper understanding and mitigation of discrimination in insurance.
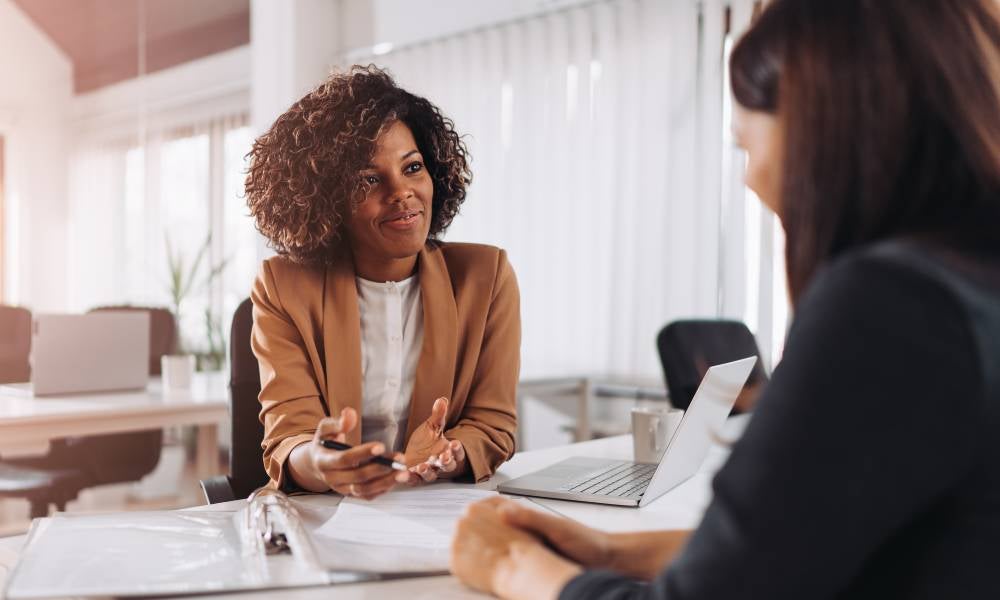
AI’s role in insurance discrimination: a grey legal area
AI and big data analytics tools are generally used on pricing and underwriting, claims management, and sales and distribution. But to date, insurers have only taken limited approaches to ensure fair and ethical outcomes in the use of AI and big data in underwriting and pricing. According to Dr Huang, as the use of AI proliferates, this will have to change. This is partly because Australia is implementing the Consumer Data Right (CDR), which will give consumers the right to share their data between service providers of their choosing. This poses opportunities, challenges, and uncertainties for insurers using data based on CDR for underwriting. But until this comes into effect, there remains a large grey regulatory area at the intersection of AI, insurance, and discrimination.
While direct discrimination is prohibited, indirect discrimination using proxies or more complex and opaque algorithms has not been specified or assessed, explains Dr Huang. “With the rapid development of AI technologies and insurers’ extensive use of big data, a growing concern is that insurance companies can use proxies or develop more complex and opaque algorithms to discriminate against policyholders,” she says.
Read more: Pricing fairness: tackling big data and COVID-19 insurance discrimination
While many insurance companies avoid using or collecting sensitive or potentially discriminatory information and argue that the output produced by analytics algorithms without using discriminatory variables is unbiased and based only on statistical evidence, indirect discrimination still occurs. “This happens when proxy variables (i.e., identifiable proxy) or opaque algorithms (i.e., unidentifiable proxy) are used. Therefore, there is an urgent need globally for insurance regulators to propose standards to identify and address the issues of indirect discrimination,” explains Dr Huang.
Some common examples of proxies include postcodes, credit information, education level, and occupation. In the US, many of these are already regulated, mainly because of their negative impact on (racial) minorities and low-income individuals. However, with the advent of AI and big data, Dr Huang says restricting or prohibiting the use of proxies alone does not mitigate all potential indirect discrimination, since some proxies are no longer easy to identify.
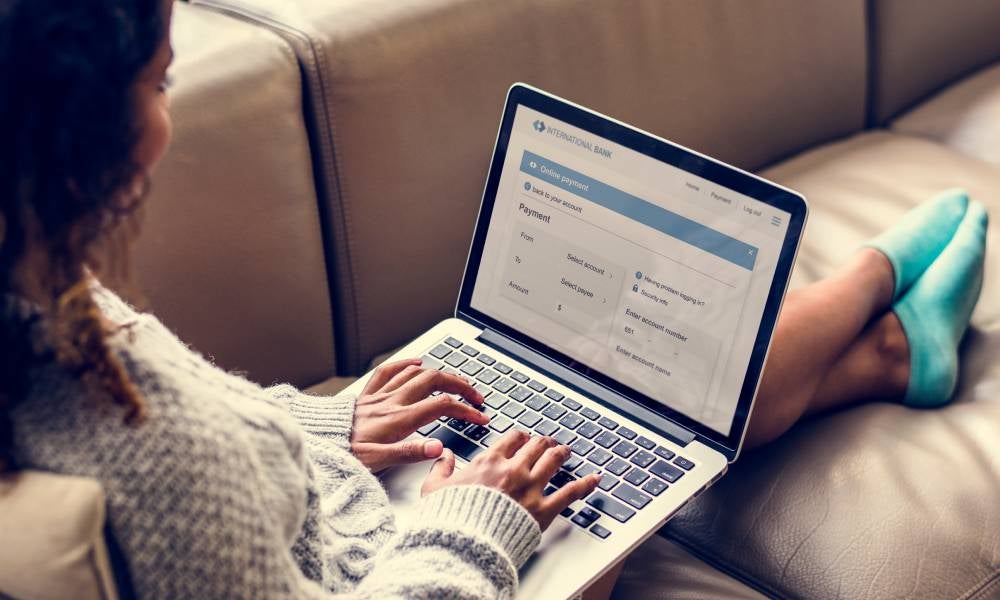
What is indirect discrimination?
In Australia, federal anti-discrimination laws cover a wide range of grounds broadly including race, sex, disability and age. While the Australian Human Rights Commission (AHRC) also has specific guidelines related to indirect discrimination, there is no clear guidance in the regulation of insurance practice, explains Dr Huang.
“After avoiding direct discrimination, indirect discrimination occurs when a person is still treated unfairly compared to another person under implicit inference from their protected characteristics, based on a neutral practice (e.g. proxy variables, opaque algorithms),” she says.
“To the extent of our knowledge, there is no existing legal framework in any jurisdiction to explicitly assess indirect discrimination in the insurance sector,” she says. But there are signs of change ahead, with the AHRC currently working with the Actuaries Institute to develop guidance for actuaries. This will be very beneficial, according to Dr Huang. But discrimination remains a complex word in the world of insurance.
“The nature of insurance is risk pooling, and the essence of pooling is discrimination, which is a business necessity for insurance companies to discriminate against insureds by classifying them into different risk pools and each pool with a similar likelihood of losses,” says Dr Huang.
Read more: How insurers skim your personal online data to price insurance
“Risk classification benefits insurers as it reduces adverse selection, and moral hazards, and promotes economic efficiency, while some consumers may worry about being unfairly discriminated against by insurance companies with more frequent use of big data and more advanced analytics tools,” she explains. “In many other fields, the term ‘discrimination’ carries a negative connotation implying that the treatment is unfair or prejudicial, while in the insurance field, it often retains its original neutral meaning as the act of distinguishing."
With the proliferation of AI and big data, the meaning has shifted yet again. While insurance companies are not allowed to use certain protected characteristics (those characteristics are usually also socially unacceptable) to directly discriminate against policyholders in underwriting or rating, such as race, religion, or national origin, they continue to discriminate indirectly.
A solution to AI bias and discrimination in insurance
To address the problem of indirect discrimination, Dr Huang’s study examines the different degrees of anti-discrimination regulations, which are reviewed in this paper from an international perspective, including the US, EU, and Australia. The study lines up different regulations on the spectrum, ranging from no regulation, restrictions (or prohibitions) on protected or proxy variables, the disparate impact standard, to community rating. They noticed that the insurance regulations varied by the line of business and jurisdiction.
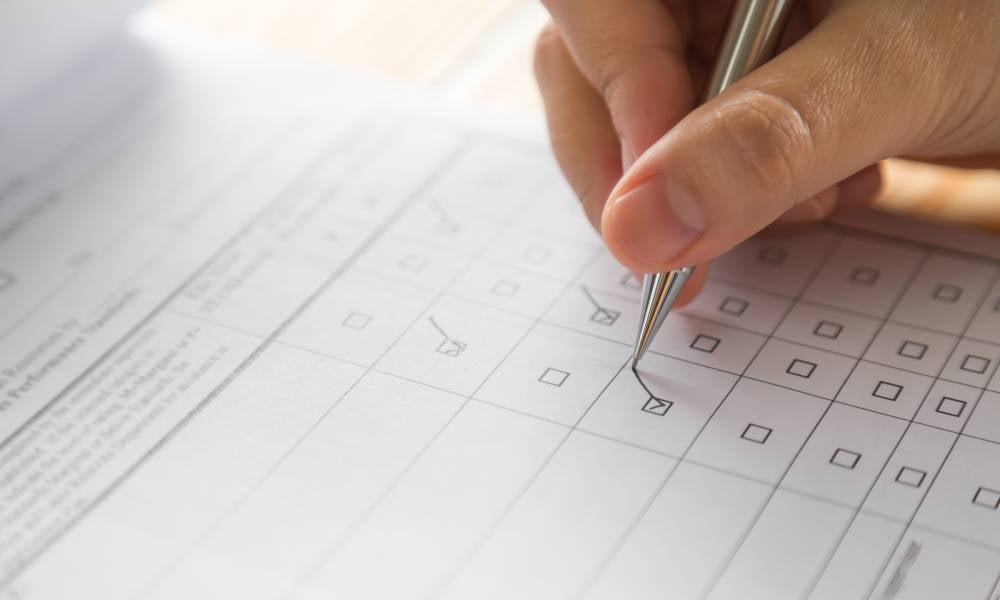
The authors then match the different regulations with fairness notions, including both individual fairness and group fairness. These fairness criteria aim to either achieve fairness at the individual or group level, noting that an inevitable conflict may exist between group fairness and individual fairness, explains Dr Huang.
Finally, the authors implement the fairness criteria into a series of existing and newly proposed anti-discrimination insurance pricing models. They compare the outcome of different insurance pricing models via the fairness-accuracy trade-off and analysed the implications of using different pricing models on customer behaviour and cross-subsidies.
How fairness criteria tackle discrimination
While changing the narrative around discrimination and insurance could be an important step, Dr Huang’s study ultimately finds that anti-discrimination models must also be based on these fairness criteria to prevent discrimination. She explains: “In our paper, we discussed four different anti-discrimination insurance pricing models corresponding to four fairness criteria to mitigate indirect discrimination. And we are studying more fairness criteria, their welfare implications, and assessment tools for regulators and insurers to use.”
Subscribe to BusinessThink for the latest research, analysis and insights from UNSW Business School
"There are three ways to mitigate indirect discrimination: pre-processing (mitigating data bias before modelling), in-processing (mitigating bias during model training), and post-processing (mitigating bias by processing the model output). Depending on specific anti-discrimination regulations, insurers could choose the appropriate strategies in practice, based on the link created between the regulation, fairness criteria, and insurance pricing models.”
Dr Huang's body of work to date highlights this problem will require actuaries to collaborate and discuss solutions with experts from multiple disciplines. “Academics and external partners from a wide range of disciplines (such as actuaries, economics, computer science, law, political science, philosophy, and sociology) need to work together to tackle difficult research problems, including algorithmic ethics and discrimination (certainly including, but not limited to insurance),” she says.
“We expect to see more structured multidisciplinary teams of this nature emerging to tackle large societal problems.”
Dr Fei Huang is a Senior Lecturer in the School of Risk & Actuarial at UNSW Business School. For more information, please contact Dr Huang directly.