Building socially intelligent AI: Insights from the trust game
As AI continues to evolve, the ability to develop socially intelligent systems will become increasingly important for organisations aiming to harness its full potential
The evolution of artificial intelligence (AI) has sparked immense interest in how these systems can emulate human behaviours, particularly in social contexts and the business world.
Customer service is one process that is already benefiting significantly from the application of AI, with the promise of enhanced interaction and satisfaction. AI systems with social intelligence, for example, can significantly improve customer service by providing more empathetic and effective interactions. For example, LivePerson uses AI-powered chatbots to enhance customer support. These chatbots understand and respond to customer emotions, offering better support and resolving issues in a way that builds trust and satisfaction. LivePerson’s AI learns from each interaction, improving its responses over time and providing a more human-like experience.
In the finance industry, trust is also a critical component of client relationships. AI systems that have learned to exhibit trust and trustworthiness can significantly improve client interactions. For instance, Wealthfront, a prominent robo-advisor, uses AI to provide personalised financial advice. Wealthfront’s algorithms consider individual client goals, risk tolerance, and market conditions to create tailored investment strategies. By simulating human-like trust behaviours, these AI systems can build stronger, more trusting relationships with clients, leading to higher satisfaction and loyalty.
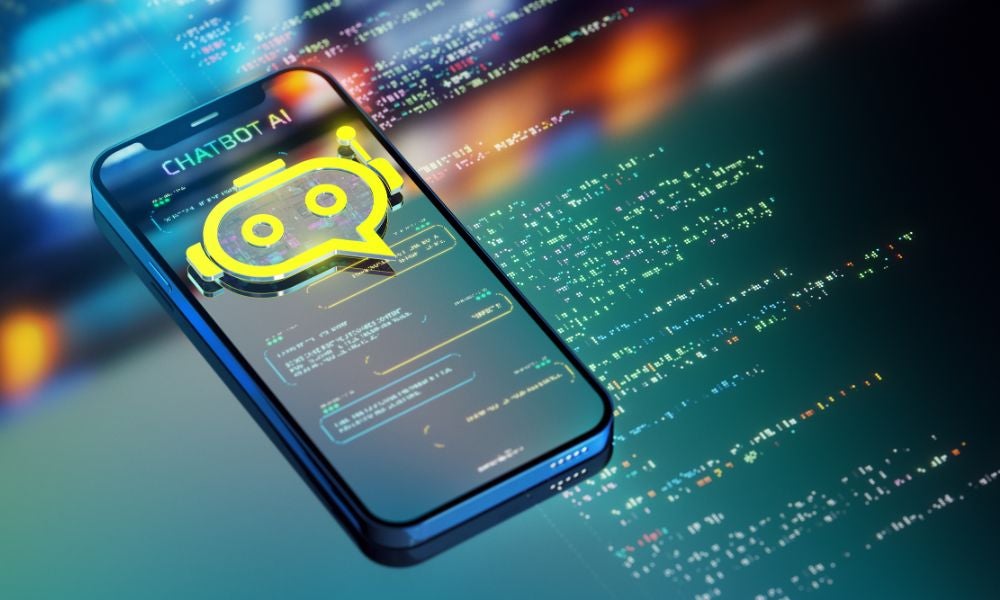
How AI agents play the trust game
A recent study, Building Socially Intelligent AI Systems: Evidence from the Trust Game Using Artificial Agents with Deep Learning, delves into this subject by examining AI agents’ performance in the trust game, a well-established measure of trust and trustworthiness. Published in Management Science, the significance of this research lies in its potential to bridge the gap between AI capabilities and human social interaction, a critical area for developing AI systems that can operate effectively in real-world environments.
The authors of the study, Dr Jason Xianghua Wu, a lecturer in the School of Information Systems and Technology Management at UNSW Business School, together with Professor Diana Yan Wu from San Jose State University, Professor Kay Yut Chen from the University of Texas at Arlington and Assistant Professor Lei Hua from the University of Texas at Tyler, highlighted the need to understand not just the technical capabilities of AI, but also how these systems can be trained to exhibit behaviours that foster trust and cooperation, essential elements in social interactions.
In their research, the authors used the trust game, a two-player economic exchange game where one player, the trustor, decides how much of a given endowment to send to the second player, the trustee. The amount sent is tripled, and the trustee then decides how much of this tripled amount to return to the trustor. The game is designed to measure the levels of trust and trustworthiness between players. In their study, the researchers used AI agents to participate in the trust game to observe whether they could develop behaviours akin to human trust and cooperation.
Read more: Explainer: How ChatGPT works in a sentence
They wanted to determine if AI agents, through learning algorithms, could reach levels of trust and cooperation typically seen in human interactions. The researchers constructed deep neural network-based AI agents using the deep-Q-network (DQN) method, a reinforcement learning algorithm. These agents played the trust game repeatedly without any prior knowledge of human behaviour. The methodology focused on evaluating if AI agents could mimic social behaviours through a trial-and-error learning process. The AI agents were trained in two roles: trustor and trustee, interacting with one another to explore the emergence of trust and cooperative behaviour.
Can AI really develop trustworthiness?
The research found that under specific conditions, AI agents could develop behaviours similar to human participants in the trust game. The AI agents were able to produce actions reflecting trust and trustworthiness purely from the interactive learning process. The research paper identified several key conditions that fostered these cooperative behaviours in AI agents, including meticulously designed training protocols, a detailed history of past actions, and well-structured incentives for future rewards.
One of the significant insights from this research is the identification of conditions that fostered cooperative behaviours in AI agents. These included the training protocols, the history of past actions, and the incentives for future rewards. For instance, the agents were more likely to exhibit trust when their training included varied scenarios that mimicked real-world complexities.
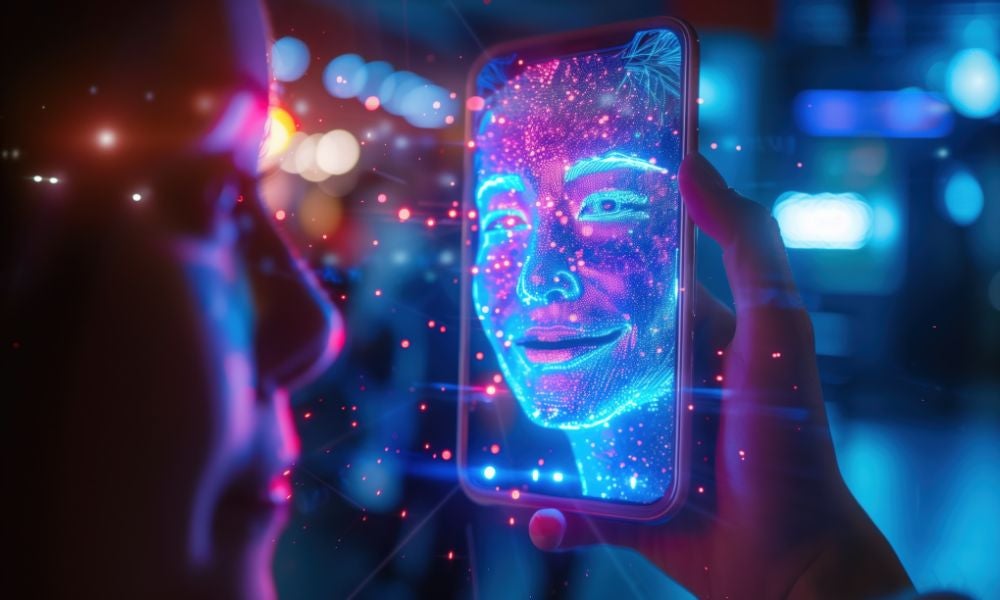
The history of past actions also played a crucial role, as AI agents could adjust their strategies based on previous experiences, much like humans. Additionally, the structure of rewards and penalties significantly influenced the development of trust and cooperation, highlighting the importance of incentive design in AI training.
The results indicate that AI systems can be designed to develop social intelligence, which is crucial for decision-support systems in various fields. Social intelligence in AI could enhance the performance of systems in areas that require nuanced understanding and interaction with humans. The ability to simulate human-like trust and cooperation means AI could potentially handle tasks that require a high level of interpersonal skills.
“We discover that deep neural network-based artificial agents can establish humanlike trusting and trustworthy behaviours by learning interactively as fixed partners. The AI agents, unlike humans, are not subject to any influence from biological or demographic differences,” the researchers said in their paper. “The resulting levels of cooperation in the trust game depend nonlinearly on the length of memory and the weight of future rewards built into DQN agents. These findings are eerily similar to our understanding of trust and trustworthiness in humans from existing literature.”
Read more: James Cameron on how AI will impact creativity and innovation
Practical applications
The implications of these findings are significant, particularly for organisations looking to integrate AI into decision-making processes. By understanding how AI can develop social intelligence, businesses can create more effective decision support systems that optimise and automate activities in fields such as finance, healthcare, cybersecurity, and supply chain management.
For example, AI systems with social intelligence can enhance collaboration between different stakeholders, including suppliers, manufacturers, and distributors. IBM’s Watson supply chain solution employs AI to predict potential conflicts, understand the priorities and constraints of each party, and facilitate negotiations that lead to mutually beneficial outcomes. For instance, Watson’s AI can negotiate delivery schedules and pricing based on real-time data and historical interactions, ensuring that all parties are satisfied and operations run smoothly. This leads to reduced delays, cost savings, and improved overall efficiency.
The ability to replicate human social behaviours in AI systems could revolutionise the way businesses operate, leading to more efficient and effective decision-making processes. AI systems equipped with social intelligence can potentially resolve complex cooperation problems that are fundamental in business and economic decision-making.
Subscribe to BusinessThink for the latest research, analysis and insights from UNSW Business School
“To instil human values in AI, many recent studies use human data to train artificial agents,” the researchers said in the paper. “We propose a different approach that does not require human interventions, as the use of training dataset can be biased. We argue that training AI agents to play games that require social interactions and contrasting them with human decision-makers could help deepen our knowledge of AI behaviours in different social contexts. Moreover, since social behaviours of AI agents can be endogenously determined through interactive learning, it may also provide a new tool for us to explore learning behaviours in response to the need for cooperation under specific decision-making scenarios.”