Understanding customer decision-making: The Amazon challenge
How AI-powered large language models transform customer decision-making analysis, offering scalable, efficient and actionable marketing insights in the process
When Amazon launched its first Kindle e-reader in 2007, the company faced a critical challenge understanding how customers made complex purchase decisions. Traditional market research methods like customer surveys and focus groups produced mixed results. Customers struggled to articulate the specific combination of features – from screen size and battery life to price point and storage capacity – that would influence their purchase decision.
The process was labour-intensive and costly. Amazon’s market research teams had to manually code and interpret thousands of customer responses, looking for patterns in how people evaluated different product features. Even with significant resources invested, the results were often inconsistent and difficult to scale across Amazon’s growing product categories.
This challenge reflected a broader issue facing businesses: how to efficiently capture and interpret customer preferences for complex products with multiple features. Companies needed a way to process large volumes of unstructured customer feedback – from product reviews to survey responses – and extract meaningful insights about decision-making patterns.
This real-world challenge provided the foundation for recent research into how artificial intelligence, specifically large language models, could transform the process of understanding customer decision-making. Published in the journal, Customer Needs and Solutions, the study Leveraging LLMs for Unstructured Direct Elicitation of Decision Rules, authored by Dr Songting Dong, Senior Lecturer in the School of Marketing at UNSW Business School, examined how large language models (LLMs) could transform the way businesses understand customer decision-making. The study addressed a critical business need: finding a more efficient, scalable way to process and interpret unstructured customer feedback while maintaining or improving accuracy compared to human analysis.
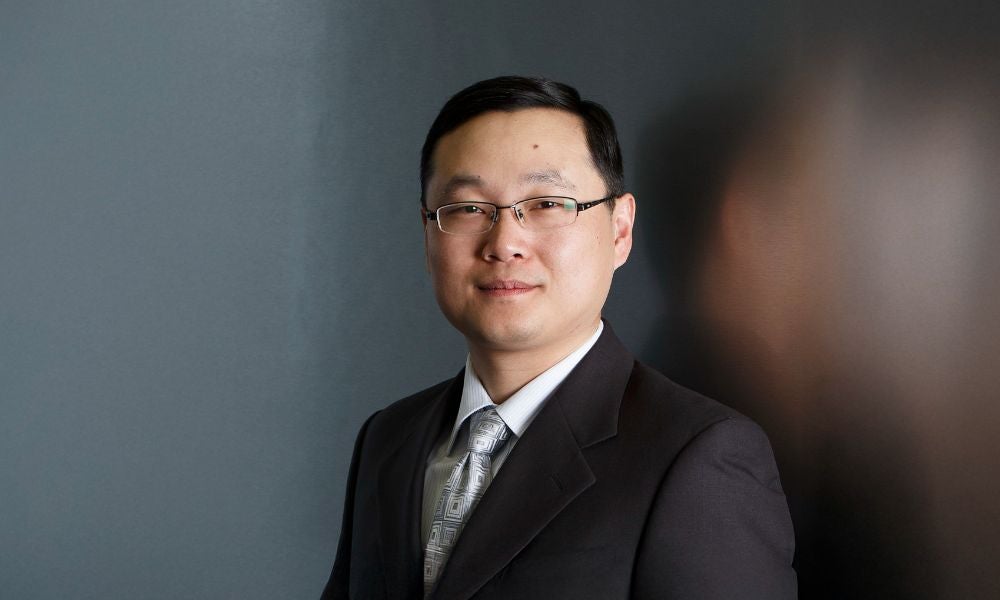
“Understanding and predicting consumer preferences and decision rules are fundamental aspects of marketing research, as they provide crucial insights into consumer behaviour and decision-making processes in various contexts,” Dr Dong explained in the research. “In today’s marketplace, eliciting consumer preferences faces new challenges as new products have become increasingly more complex, and consumers continuously generate reviews, comments, and other forms of feedback online.”
Understanding customer decision-making at scale
The research investigated how effectively LLMs could interpret customer preferences compared to traditional human analysis. The study investigated two major experiments – one focused on automotive purchases and another on mobile phones – involving hundreds of customer emails describing their decision-making processes.
The results demonstrated significant potential for AI-powered analysis. In the automotive study findings, for example, Dr Dong noted that finetuned LLMs effectively interpret decision rules and handle sophisticated considerations in a complex product scenario, outperforming the best unstructured direct elicitation models by capturing over 25% more information.
The research was inspired by the pressing challenges businesses face in decoding consumer preferences for increasingly complex products, Dr Dong explained. “Traditional methods like customer surveys and focus groups often fell short in capturing the nuanced and dynamic decision-making processes of modern consumers, particularly as products became more feature-rich and customer feedback increasingly unstructured,” he said.
Read more: AI-powered marketing: From the classroom to the boardroom
This gap sparked the idea to explore whether advanced AI, particularly LLMs, could offer a scalable and efficient alternative. “The goal was to evaluate whether LLMs could not only replicate but also surpass human analysis in interpreting customer decision rules, providing deeper insights and improving predictive accuracy across various contexts,” he said.
Additionally, with the unique ability of finetuned LLMs to learn from existing text and interact with users, Dr Dong said these models are not only capable of summarising consumer preferences and decision rules but also of supplying actionable insights for businesses. By leveraging their capacity to process and interpret unstructured customer feedback, Dr Dong said finetuned LLMs can assist in the creation and simulation of marketing strategies tailored to specific consumer segments. “This capability positions LLMs as invaluable tools for marketers, enabling the design of data-driven strategies with greater precision and scalability,” he said
The power of AI in complex decision analysis
One of the most promising findings was how well LLMs performed with complex products involving multiple features and decision factors. The research found that as problem complexity increases and consumer input becomes longer and harder to interpret, unstructured direct elicitation models struggle more than LLMs. In such complex environments, Dr Dong explained that finetuned LLMs are better equipped to extract decision rules and maintain higher predictive performance.”
The contrast between simple and complex decision scenarios was particularly revealing. In the mobile phone study, which involved fewer variables, traditional analysis methods performed adequately. However, when faced with the complexity of automotive purchase decisions, where customers had to evaluate multiple interconnected features, the advantages of LLMs became clear.
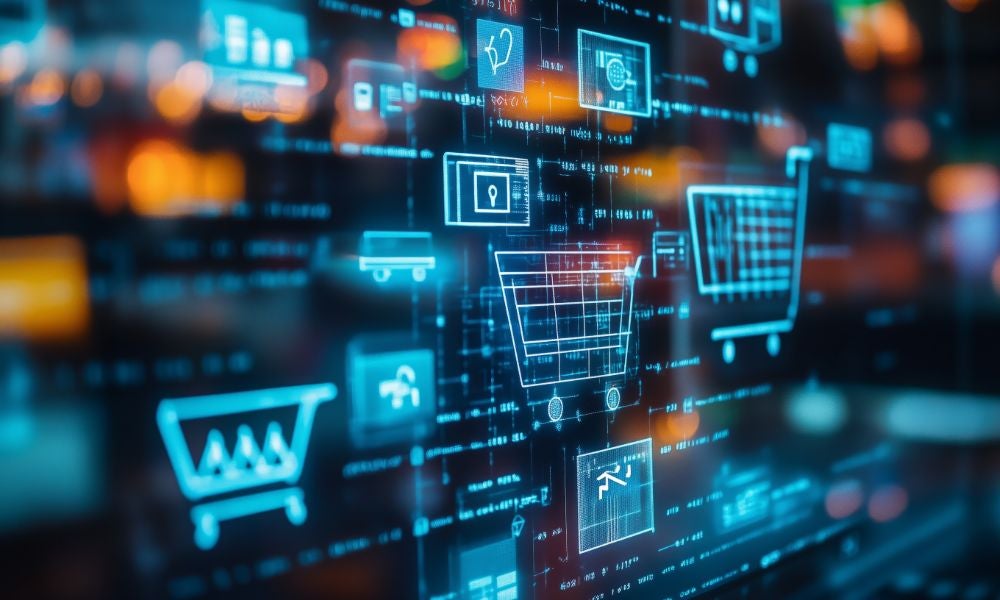
The research revealed that customer emails in the automotive study were nearly twice as long as those in the mobile phone study, averaging 1213 words compared to 626 words. This increased complexity presented a significant challenge for traditional analysis methods. “Given the increased complexity, the amount of information that models are able to capture indeed drops,” Dr Dong explained in the research paper. However, the LLMs demonstrated remarkable resilience. While finetuned LLMs captured 30.6% of the information in the mobile phone study’s initial validation set, they still maintained 17.2% information capture in the more complex automotive study.
This performance gap became even more pronounced when compared to traditional methods. “In contrast, the best unstructured direct elicitation model experienced a sharper decline in performance,” the research noted. Traditional models captured only 13.7% of the information in the automotive study, representing just 79.7% of the LLM’s performance. These findings suggest that as products and services become more complex, the advantage of using LLMs for customer preference analysis grows significantly.
Practical applications for business
The research highlighted several key advantages of using LLMs for customer preference analysis. “These advantages position LLMs, particularly finetuned LLMs, as good candidates for replacing human agents and implementing the unstructured direct elicitation method in real-world applications with better predictive power, cost efficiency, timing and scalability,” Dr Dong explained in the research paper.
While the technology still has limitations, its ability to process complex, unstructured customer feedback more effectively than human analysts suggests significant potential for improving customer insight processes across industries. For business professionals looking to implement these findings, the research suggested starting with a smaller dataset to finetune the LLM before scaling up. The study found that training on just 25% of customer responses was sufficient to achieve strong results.
Subscribe to BusinessThink for the latest research, analysis and insights from UNSW Business School
The research offers several practical implications for business professionals. Dr Dong said one key takeaway is the potential for finetuned LLMs to significantly enhance the efficiency and accuracy of customer insights processes. “By leveraging LLMs, businesses can reduce reliance on manual coding and human analysis, saving time and resources while achieving more consistent results,” he said.
“For practitioners, starting with a smaller, representative dataset to finetune an LLM before scaling up is a practical approach to implement this technology. Additionally, the interactive capabilities of finetuned LLMs allow them to serve as dynamic knowledge bases, offering not only predictions but also explanations for consumer behaviour. This interpretability can support more informed decision-making, enabling businesses to design and refine marketing strategies that resonate with target audiences in a rapidly evolving marketplace.”
However, the research also noted important limitations. “Although the frequency of hallucinations may decrease as LLMs continue to evolve, no current architecture has effectively eliminated this issue,” Dr Dong cautioned. “Therefore, I recommend that users treat LLM outputs as exploratory findings rather than empirical conclusions. Diligently checking LLM outputs and consolidating or cross-checking them with other forms of marketing research can enhance their reliability and ensure that insights derived from these models are both accurate and actionable,” he concluded.