How businesses can overcome AI adoption challenges
How can organisations best navigate AI adoption challenges, such as data quality and ethical concerns, while leveraging pilot projects for AI innovation?
While most organisations see the potential benefits of implementing AI into their businesses, many are still in the early stages of AI adoption. For example, Open AI has 250 million active weekly users versus 5.5 billion active internet users. For non-tech companies, it can be difficult to fully embrace AI for a number of reasons, according to Daniel Lavecky, a seasoned entrepreneur and fintech pioneer who holds multiple roles, including Managing Partner of Double Bay Capital, an investment firm focusing on disruptive fintech and technology ventures.
These reasons include a lack of internal AI-skilled staff, a clear understanding of how to effectively leverage AI for their specific business, concerns about accuracy, intellectual property protection risks, and a lack of regulatory guardrails. Mr Lavecky observed that many organisations are starting with small-scale pilot projects to test AI's capabilities and assess its potential impact. “For example, Microsoft just released a paper on a six-month trial of Copilot by approximately 5800 government employees,” said Mr Lavecky, who recently spoke at the UNSW Management Innovation Conference.
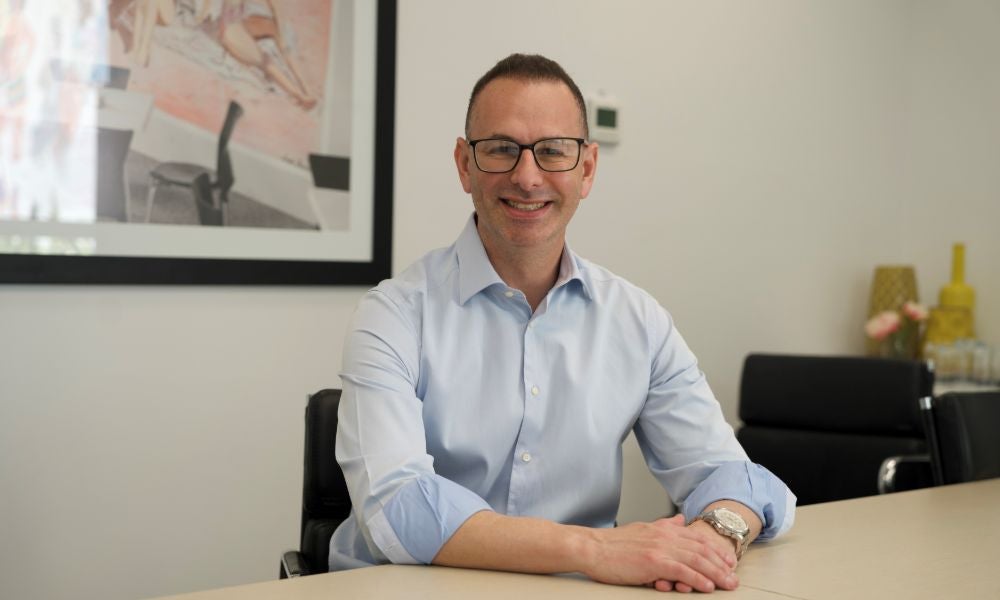
The most used AI features in the pilot were meeting summaries, summarisation, and rewriting suggestions of emails and documents, while content generation, task management and formula analysis AI tools had low adoption. “This may be because employees didn’t really understand the benefits of the AI tool and that it was a general AI and wasn’t specific to the government department,” said Mr Lavecky. “However, the use of small-scale pilots allows organisations to gain experience, build internal expertise, and mitigate risks before scaling up their AI initiatives.”
Common AI challenges and issues
Mr Lavecky, who also serves as a Guest Lecturer at AGSM @ UNSW Business School, said the most common challenges for businesses in implementing AI systems include data quality and accessibility. “An AI model is only as good as the information it is fed,” said Mr Lavecky. Many organisations struggle with data quality issues, data silos across departments and even staff, as well as concerns about data privacy, and he pointed out that IT companies like Atlassian have recently released a service called Rovo to unlock organisational knowledge wherever its located.
A significant concern about data privacy involves internal company users of an AI system who potentially gain access to information that was not intended for them. In the Microsoft Copilot Government trial, for example, Mr Lavecky said Copilot exposed improperly stored or even “classified” documents, raising significant data governance and privacy issues. “This highlights the need for organisations to implement strict access controls, data classification protocols, and audit trails, especially in highly regulated industries such as finance and healthcare,” he said.
Read more: Regulating AI in Australia: Challenges and opportunities
AI also raises ethical concerns related to bias, fairness, transparency and accountability. “Organisations need to develop robust ethical frameworks and guidelines to ensure that their AI systems are used responsibly and ethically both for internal work and when dealing with customers,” said Mr Lavecky. Elon Musk, for example, recently criticised Google's Gemini AI chatbot for its response to a question on “whether it is valid to misgender Caitlyn Jenner to prevent a nuclear apocalypse.” Mr Lavecky said this incident underscores the potential dangers of AI systems reflecting and amplifying human biases.
“We usually do not know how AI makes decisions and the extent to which it can be trusted to consistently make ethical and good decisions, and whether it can make expedient good decisions when necessary,” added Chris Jackson, Professor of Business Psychology in the School of Management and Governance at UNSW Business School and an organiser of the conference. “We need to be careful in giving AI too much power.”
What are successful "early AI adopters" doing?
Many organisations can learn from early adopters within the corporate area who have been implementing AI in various departments to streamline operations, enhance decision-making, and improve customer experiences.
“It is not surprising that tech teams are early adopters and are using AI to improve staff productivity by using AI to assist with writing code 10 times faster than by themselves,” said Mr Lavecky. “In addition, the code can be reviewed by the AI to suggest improvements, determine security vulnerabilities and help with bug fixing.”
Another area that could be considered an early adopter is the field of customer service. “Although in its infancy, we are now starting to see customer service departments (such as those at Commonwealth Bank) explore how to use chatbot and call centre AI agents that listen to calls and provide the operator with suggested answers based on previous calls as well as real-time information about the particular client,” said Mr Lavecky. “The AI agents have access to corporate data, documents and processes, and are able to support the customer to gain a better customer service experience and hopefully resolution.”
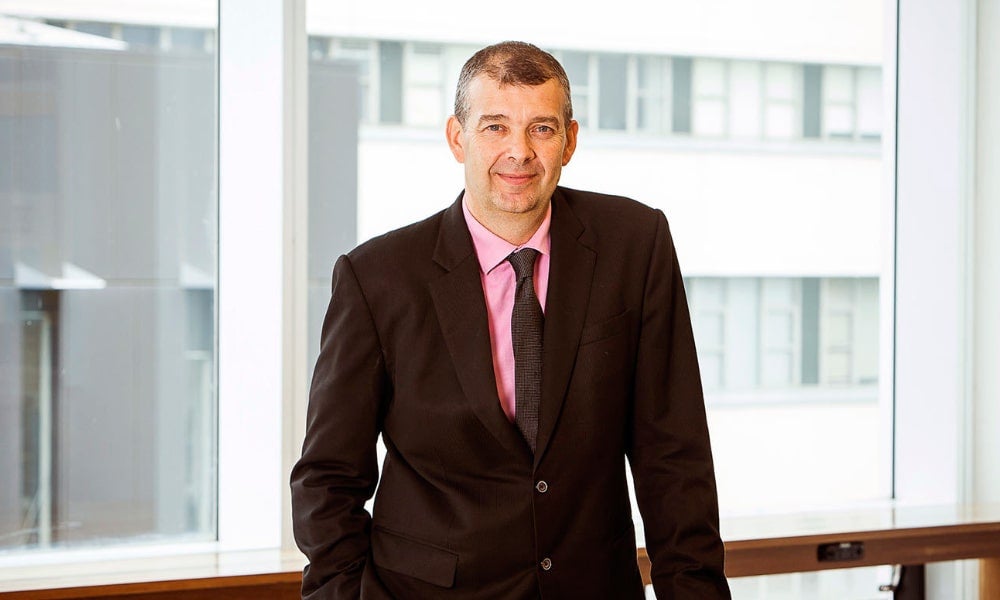
“Mr Lavecky identifies the early adopters of AI but there is a full range of people from early adopters to those who will resist change to the bitter end,” Prof. Jackson added. “It’s good to discover who these late adopters are in an organisation and offer them assistance.”
Future fintech and technology trends
Mr Lavecky also shared predictions with the audience about trends that may disrupt the fintech industry in the future. One of the more significant trends involves AI-driven fraud detection and risk management. “Machine learning models analyse huge volumes of transactions concurrently, determining patterns in real time, flagging unusual activity to prevent fraud,” said Mr Lavecky, who shared an example from another firm where he serves as Executive Chairman: CANVAS Blockchain Group, which connects investors, issuers and blockchain-based assets through a regulated, institutional-grade gateway to Web3.
“At Canvas, we use AI agents to detect and mitigate fraud at scale, improving security while reducing false positives that hinder customer experience,” he explained. “These AI agents can adjust to economic fluctuations dynamically, allowing us to make better-informed decisions. Additionally, in the highly regulated financial industry, AI can detect discrepancies and anomalies in data that might indicate compliance breaches or risky practices, allowing financial firms to identify and address issues in real-time.”
Subscribe to BusinessThink for the latest research, analysis and insights from UNSW Business School
Another important trend involves the continued tokenisation of real-world assets. “Blockchain technology at my company, Canvas, enables the tokenisation of real-world assets such as bonds, real estate, managed funds and art, so that ownership is represented with a token,” he said. “Like digitisation of publicly traded shares in the 80s, tokenisation creates new financial markets and opportunities for liquidity, plus the ability to trade 24/7.”
This trend, combined with the rise of Central Bank Digital Currency (CBDCs), is poised to disrupt traditional financial institutions, Mr Lavecky said. It also has the potential to create financial products and services “that we have not seen yet” in combination with some other AI-driven financial market uses.
A third important trend revolves around AI-driven predictive analytics for portfolio management and optimisation. “AI is being used to churn through huge amounts of data to analyse past performance, market trends and predict future trends and risks,” said Mr Lavecky. “Financial institutions like JP Morgan are now trying to make more informed investment and credit decisions. Firms like BlackRock leverage AI to manage large portfolios, assessing risk with high precision based on data-driven insights and historical patterns. This potentially can be rolled out to the everyday investor in the future.”